Transform data
Move or enrich data from a source system, between data models, or from the Cognite Data Fusion (CDF) staging area into CDF by transforming data into CDF's data model or user-defined data models. You can also transform data to the CDF staging area for further preparations.
You can set a schedule for transformation runs and monitor transformation by activating automatic email notifications.
You can also transform data using the Cognite API, the Cognite Python SDK, and the Cognite Toolkit.
Before you start
Make sure you've completed these steps to register an app for the transformation in your identity provider (IdP) and to set up the necessary folders and capabilities to run or schedule transformations.
The data you want to transform must conform to the structure defined by the data model.
Step 1: Create a transformation
-
Navigate to Data management > Integrate > Transformations.
-
Select Create, and enter a unique name and a unique external ID.
-
Optionally, associate the transformation to an existing data set to restrict who can access the transformation.
-
Select Next.
-
Select the target data model that contains the target resource type.
- CDF resource types - select this model to ingest data into an asset hierarchy.
- If you're ingesting data into the Assets resource type, make sure a parent asset already exists in CDF.
- If you're ingesting data into Sequence rows, specify the external ID for the sequence you want to write to. This defines the target schema.
- CDF staging area - select this model to ingest data into the CDF staging area. You must specify the target database and table.
- User defined data models - select this model to ingest data in a user defined data model. Enter the target space you want to write data to and the version of the data model. CDF sets the default space from the data model. You can ingest data into a type or a relationship.
- CDF resource types - select this model to ingest data into an asset hierarchy.
-
Under Action, select if you want to create, update, delete, or create or update the target data. If you're updating existing data, you must specify how the transformation should set null values:
-
Select Keep existing values to not update existing data. This is the default setting.
-
Select Clear existing values to set existing values to null, for example, when a piece of equipment is removed for maintenance. Use this option to disassociate the asset from its parent in the asset hierarchy.
-
To delete existing rows in a CDF staging table, you must use the Transformations API.
Step 2: Map source and target data
In the editor pane, you can create transformations with the mapping editor or enter Spark SQL queries. Typically, you would use the mapping editor to copy data from source to target resource types and use SQL queries to perform more complex transformations.
Using the mapping editor
-
Select the source table and map source fields to the target fields. Required fields are marked with an
!
next to the field name.tipIf you're unsure about the mappings, select View suggestions to get help. This is especially useful for larger data types with many attributes.
-
Select Preview to verify that the transformation produces the expected output.
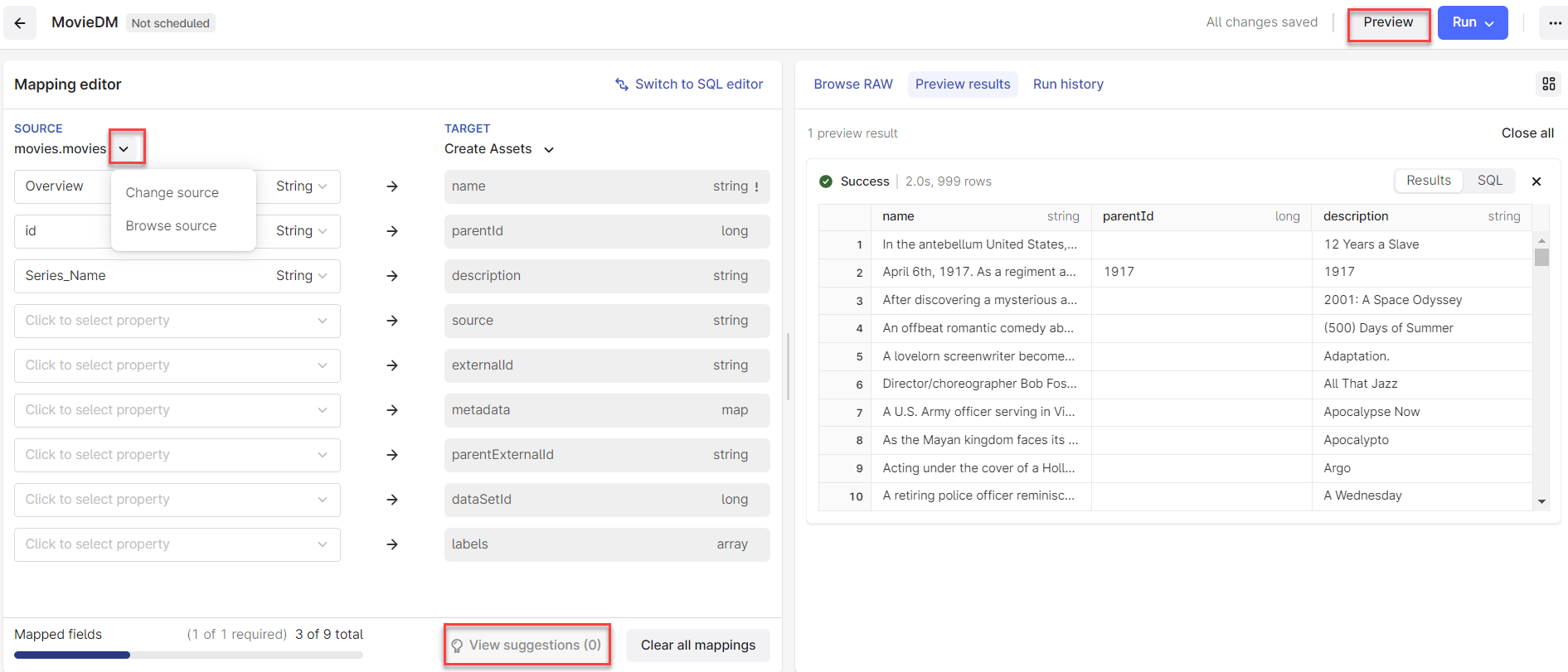
Using Spark SQL
Select Switch to SQL editor to create a transformation in Spark SQL. The Spark SQL editor offers built-in code completion and built-in Spark SQL functions and Cognite custom SQL functions.
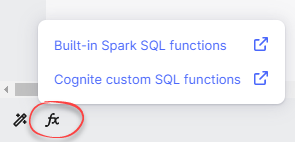
Your changes won't be kept if you switch from the SQL editor to the mapping editor.
Step 3: Transform data
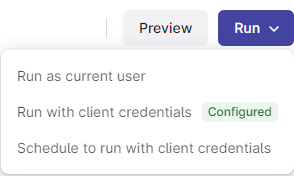
Select Run to start a transformation, or follow the steps in schedule transformations to run your transformation at regular intervals.
Select Run with client credentials and specify Client ID and the Client secret for the app you registered for the transformation in Microsoft Entra ID. CDF automatically refills the remaining fields.
You can also select Run as current user when you want to run one-time transformations, for instance, to create the root node in an asset hierarchy, create a new CDF RAW table from other RAW tables, or add data manually. We recommend using Run with client credentials.
Add the capability sessions:create
to enable the Run as current user option.
Select the Advanced authentication method to specify separate credentials for reading and writing data. For instance, if you want to transform data between different projects.
If you don't know what values to enter in these fields, contact your internal help desk or the CDF admin for help.
To edit credentials, schedules, and notifications for a transformation, navigate to More options (...) at the top of the page.
Step 4: Schedule transformations
-
Select Run > Set schedule.
-
The first time you schedule a transformation, you must enter your Client ID and the Client secret.
-
Specify when and how often you want the transformation should run. Select a predefined schedule or specify a cron expression.
For example,
45 23 * * *
will run the transformation at 23:45 (11:45 PM) every day. -
Select Set schedule to activate the schedule. CDF sets the transformation to read-only to prevent unintentional changes to future scheduled jobs.
CDF will block transformations from running after five consecutive failed runs or if the client credentials have changed or expired. Re-enter the read or write credentials or contact your CDF admin.
Step 5: Monitor transformations
To monitor the transformation process and solve any issues before they reach the data consumer, you can subscribe to email notifications if a transformation fails.
- Navigate to the More options (...) and select Monitor.
- Enter email addresses that will receive notifications if this transformation fails.