About contextualization
The interactive contextualization tools in Cognite Data Fusion (CDF) let you combine machine learning, a powerful rules engine, and domain expertise to map resources from different source systems to each other in the CDF data model.
This way of connecting information allows you to build applications where you, for example, can select a component in a 3D model to see all the connected time series data or ask for all the pressure readings along a flow line.
The first part of contextualization is to ensure that each unique entity shares the same identifier in CDF, even if it has different IDs in the source systems. This step is mostly performed during the transformation stage when you shape and match incoming data and compare it with existing resources in your collection.
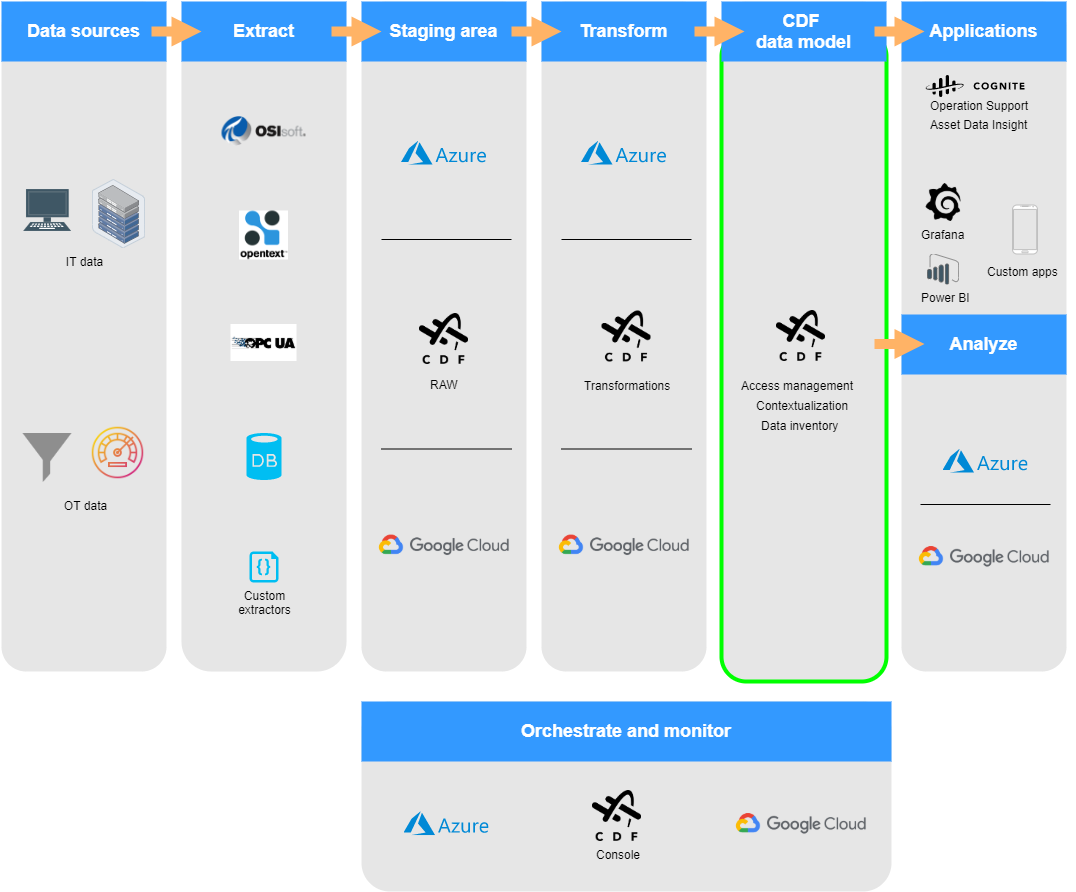
The next step in the contextualization process is associating entities with each other in the same way they relate in the real world. For instance, an object inside a 3D model may have an ID you can map to an asset, and a time series from an instrument monitoring system can have another ID you can assign to the same asset.
The contextualization tools help you build interactive engineering diagrams/P&IDs (Piping and Instrumentation Diagrams) from static PDF source files. You can find belong-to relationships between resources to set up, automate and validate all your contextualization pipelines from your browser without having to write any code.
Entity matching
Contextualize your data by using machine learning and rules engines. Then let domain experts validate and fine-tune the results.
Learn more: Entity matching
Contextualize engineering diagrams
Extract information from engineering diagrams such as static P&IDs (Piping and Instrumentation Diagrams) to create fully contextualized and interactive diagrams that you can navigate on any device.
Learn more: Contextualize engineering diagrams